On a fall morning in 2010, sitting at the kitchen table of her Illinois home, Safiya Umoja Noble plugged a pair of words into Google. She was getting ready for a sleepover with her fourteen-year-old stepdaughter, whom she likes to call her bonus daughter, as well as five young nieces. Wanting to nudge them away from their cellphones, but wary that the girls could head straight for her laptop, Noble checked to see what they might find. “I thought to search for ‘black girls,’ because I was thinking of them—they were my favourite group of little black girls,” she says. But that innocuous search instantly resulted in something alarming: a page filled with links to explicit porn. Back then, anyone searching for black girls would have found the same. “It was disappointing, to say the least, to find that almost all the results of racialized girls were primarily represented by hypersexualized content,” says Noble, now a communications professor at the University of Southern California. “I just put the computer away and hoped none of the girls would ask to play with it.”
Listen to an audio version of this story
For more Walrus audio, subscribe to AMI-audio podcasts on iTunes.
Around the same time, in another part of the country, computer scientist Joy Buolamwini discovered a different problem of representation. The Canada-born daughter of parents from Ghana, she realized that advanced facial-recognition systems, such as the ones used by IBM and Microsoft, struggled hard to detect her dark skin. Sometimes, the programs couldn’t tell she was there at all. Then a student at the Georgia Institute of Technology, she was working on a robotics project, only to find that the robot, which was supposed to play peekaboo with human users, couldn’t make her out. She completed the project by relying on her roommate’s light-skinned face. In 2011, at a startup in Hong Kong, she tried her luck with another robot—same result. Four years later, as a graduate researcher at MIT, she found that the latest computer software still couldn’t see her. But when Buolamwini slipped on a white mask—the sort of novelty item you could buy at a Walmart for Halloween—the technology worked swimmingly. She finished her project coding in disguise.
Facial recognition and search engines are just two feats of artificial intelligence, the scientific discipline that trains computers to perform tasks characteristic of human brains involving, among other things, math, logic, language, vision, and motor skills. (Intelligence, like pornography, resists easy definition; scientists and engineers haven’t honed in on a single, common-use description, but they know it when they see it.) Self-driving cars may not quite be ready to prowl city streets, but virtual assistants, such as Alexa, are all set to book a midday meeting at that coffee place you like. Improvements in language processing mean that you can read a translated Russian newspaper article in English on your phone. Recommender systems are terrifically adept at selecting music geared specifically to your taste or suggesting a Netflix series for you to binge over a weekend.
These aren’t the only areas where AI systems step in to make assessments that affect our lives. In some instances, what’s at stake is only our time: when you call for support from a bank, for example, your placement on the wait-list may not be sequential but contingent on your value to it as a customer. (If the bank thought your investment portfolio was more promising, you might only be on hold for three minutes, instead of eleven.) But AI also increasingly influences our potential employment, our access to resources, and our health. Applicant-tracking systems scan resumés for keywords in order to shortlist candidates for a hiring manager. Algorithms—the set of instructions that tell a computer what to do—currently evaluate who qualifies for a loan and who is investigated for fraud. Risk-prediction models, including the one used in several Quebec hospitals, identify which patients are likeliest to be readmitted within forty-five days and would benefit most from discharge or transitional care.
AI informs where local policing and federal security are headed as well. In March 2017, the Canada Border Services Agency announced it would implement facial-matching software in its busiest international airports; kiosks in several locations, from Vancouver to Ottawa to Halifax, now use the system to confirm identities with passports and, according to a Government of Canada tender, “provide automated traveller risk assessment.” Calgary police have used facial recognition to compare video surveillance with mug shots since 2014, and last fall, the Toronto Police Services Board declared it would put part of its $18.9 million Policing Effectiveness and Modernization grant toward implementing similar technology. And while traditional policing responds to crimes that have actually occurred, predictive policing relies on historical patterns and statistical modelling, in part to forecast which neighbourhoods are at a higher risk of crime and then direct squad cars to those hot spots. Major US jurisdictions have already rolled out the software, and last summer, Vancouver became the first Canadian city to do the same.
These technologies are prized for their efficiency, cost-effectiveness, and scalability—and for their promise of neutrality. “A statistical system has an aura of objectivity and authority,” says Kathryn Hume, the vice-president of product and strategy for Integrate.ai, an AI-focused startup in Toronto. While human decision-making can be messy, unpredictable, and governed by emotions or how many hours have passed since lunch, “data-driven algorithms suggest a future that’s immune to subjectivity or bias. But it’s not at all that simple.”
Artificial intelligence may have cracked the code on certain tasks that typically require human smarts, but in order to learn, these algorithms need vast quantities of data that humans have produced. They hoover up that information, rummage around in search of commonalities and correlations, and then offer a classification or prediction (whether that lesion is cancerous, whether you’ll default on your loan) based on the patterns they detect. Yet they’re only as clever as the data they’re trained on, which means that our limitations—our biases, our blind spots, our inattention—become theirs as well.
Earlier this year, Buolamwini and a colleague published the results of tests on three leading facial-recognition programs (created by Microsoft, IBM, and Face++) for their ability to identify the gender of people with different skin tones. More than 99 percent of the time, the systems correctly identified a lighter-skinned man. But that’s no great feat when data sets skew heavily toward white men; in another widely used data set, the training photos used to make identifications are of a group that’s 78 percent male and 84 percent white. When Buolamwini tested the facial-recognition programs on photographs of black women, the algorithm made mistakes nearly 34 percent of the time. And the darker the skin, the worse the programs performed, with error rates hovering around 47 percent— the equivalent of a coin toss. The systems didn’t know a black woman when they saw one.
Buolamwini was able to calculate these results because the facial-recognition programs were publicly available; she could then test them on her own collection of 1,270 images, made up of politicians from African and Nordic countries with high rates of women in office, to see how the programs performed. It was a rare opportunity to evaluate why the technology failed in some of its predictions.
But transparency is the exception, not the rule. The majority of AI systems used in commercial applications—the ones that mediate our access to services like jobs, credit, and loans— are proprietary, their algorithms and training data kept hidden from public view. That makes it exceptionally difficult for an individual to interrogate the decisions of a machine or to know when an algorithm, trained on historical examples checkered by human bias, is stacked against them. And forget about trying to prove that AI systems may be violating human rights legislation. “Most algorithms are a black box,” says Ian Kerr, Canada Research Chair in ethics, law, and technology. In part, that’s because companies will use government or trade-secrets laws to keep their algorithms obscure. But he adds that “even if there was perfect transparency by the organization, it may just be that the algorithms or AI are unexplainable or inscrutable.”
“People organized for civil rights and non-discriminatory lending practices and went to court under the protections of the law to try and change those practices,” says Noble, who recently published a book called Algorithms of Oppression. “Now we have similar kinds of decision-making that’s discriminatory, only it’s done by algorithms that are difficult to understand—and that you can’t take to court. We’re increasingly reduced to scores and decisions by systems that are very much the product of human beings but from which the human being is increasingly invisible.”
If you want to build an intelligent machine, it’s not a bad idea to start by mining the expertise of an intelligent person. Back in the 1980s, developers made an early AI breakthrough with so-called expert systems, in which a learned diagnostician or mechanical engineer helped design code to solve a particular problem. Think of how a thermostat works: it can be instructed through a series of rules to keep a house at a designated temperature or to blast warm air when a person enters the room. That seems pretty nifty, but it’s a trick of those rules and sensors—if [temperature drops below X] then [crank heat to Y]. The thermostat hasn’t learned anything meaningful about cold fronts or after-work schedules; it can’t adapt its behaviour.
Machine learning, on the other hand, is a branch of artificial intelligence that teaches a computer to perform tasks by analyzing patterns, rather than systematically applying rules it’s been given. Most often, that’s done with a technique called supervised learning. Humans aren’t off the hook yet: a programmer has to assemble her data, known as the inputs, and assign it labels, known as the outputs, so the system is told what to look for.
Say our computer scientist would like to build some sort of fruit salad object-recognition system that separates strawberries (a worthy addition to any fruit salad) from bananas (utter waste of space). She needs to select features—let’s go with colour and shape—that are highly correlated with the fruit so the machine can distinguish between them. She labels images of objects that are red and round strawberries and those that are yellow and long bananas, and then she writes some code that assigns one value to represent colour and another to characterize shape. She feeds a mess of photos of strawberries and bananas into the machine, which builds up an understanding of the relationship between these features, enabling it to make educated guesses about what kind of fruit it’s looking at.
The system isn’t going to be especially good when it starts out; it needs to learn from a robust set of examples. Our supervisor, the computer scientist, knows that this particular input is a strawberry, so if the program selects the banana output, she penalizes it for a wrong answer. Based on this new information, the system adjusts the connection it made between features in order to improve its prediction the next time. Quickly—because a pair of outputs isn’t much of a sweat—the machine will be able to look at a strawberry or a banana it hasn’t seen and accurately identify it.
“Some things are easy to conceptualize and write software for,” says Graham Taylor, who leads the machine-learning research group at the University of Guelph. But maybe you want a system capable of recognizing more complex objects than assorted fruit. Maybe you want to identify a particular face among a sea of faces. “That’s where deep learning comes in,” Taylor says. “It scales to really large data sets, solves problems quickly, and isn’t limited to the knowledge of the expert who defines the rules.”
Deep learning is the profoundly buzzy branch of machine learning inspired by the way our brains work. Put very simply, a brain is a collection of billions of neurons connected by trillions of synapses, and the relative strength of those connections—like the one between red and red fruit, and the one between red fruit and strawberry—becomes adjusted through learning processes over time. Deep-learning systems rely on an electronic model of this neural network. “In your brain, neurons send little wires that carry information to other neurons,” says Yoshua Bengio, a Montreal computer scientist and one of the pioneers of deep learning. The strength or weakness of the signal between a pair of neurons is known as the synaptic weight: when the weight is large, one neuron exerts a powerful influence on another; when it’s small, so is the influence. “By changing those weights, the strength of the connection between different neurons changes,” he says. “That’s the clue that AI researchers have taken when they embarked on the idea of training these artificial neural networks.”
And that’s one way AI can advance from sorting strawberries and bananas to recognizing faces. A computer scientist supplies the labelled data—all those particular faces attached to their correct names. But rather than requiring she tell it what features in the photographs are important for identification, the computer extracts that information entirely on its own. “Your input here is the image of the face, and then the output is the decision of who that person is,” Taylor says.
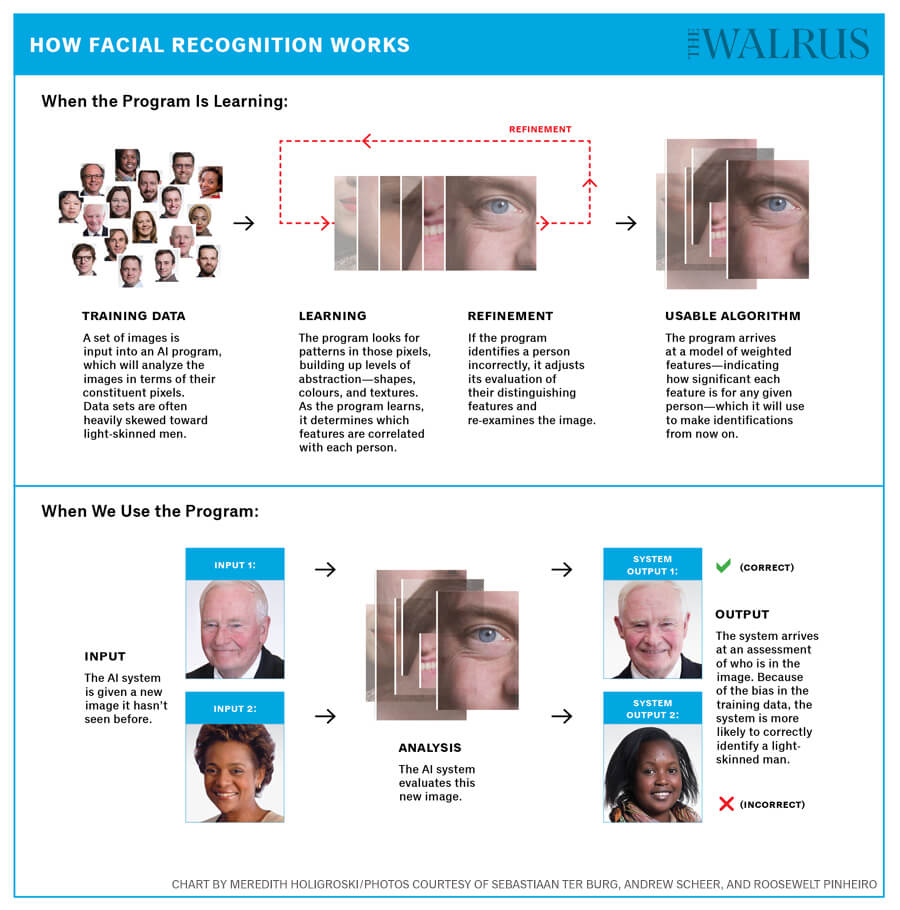
In order to make the journey from input to output, the image undergoes several transformations. “It might be transformed first into a very low-level representation, just enumerating the types of edges and where they are,” he says. The next representation might be corners and the junctions of those edges, then patterns of edges that make up a shape. A couple of circles could turn out to be an eye. “Each layer of representation is a different level of abstraction in terms of features,” Taylor explains, “until you get to the very high-level features, things that are starting to look like an identity—hairstyle and jawline—or properties like facial symmetry.”
How does this whole process happen? Numbers. A mind-boggling quantity of numbers. A facial-recognition system, for example, will analyze an image based on the individual pixels that make it up. (A megapixel camera uses a 1,000-by-1,000-pixel grid, and each pixel has a red, green, and blue value, an integer between zero and 255, that tells you how much of the colour is displayed.) The system analyzes the pixels through those layers of representation, building up abstraction until it arrives at an identification all by itself.
But wait: while this face is clearly Christopher Plummer, the machine thinks it’s actually Margaret Trudeau. “The model does very, very poorly in the beginning,” Taylor says. “We can start by showing it images and asking who’s in the images, but before it’s trained or done any learning, it gets the answer wrong all the time.” That’s because, before the algorithm gets going, the weights between the artificial neurons in the network are randomly set.
Through a gradual process of trial and error, the system tweaks the strength of connections between different layers, so when it is presented with another picture of Christopher Plummer, it does a little better. Small adjustments give rise to slightly better connections and slightly lower rates of error, until eventually the system can identify with high accuracy the correct face. It’s this technology that allows Facebook to alert you when you’ve been included in a picture, even if you remain untagged. “The cool thing about deep learning is that we can extract everything without having someone say, ‘Oh, these features are useful for recognizing a particular face,’” Taylor says. “That happens automatically, and that’s what makes it magic.”
Here’s a trick: type CEO into Google Images and watch as you conjure an array of barely distinguishable white male faces. If you’re in Canada, you’ll see sprinkled among them a handful of mostly white women, a few people of colour, and Wonder Woman’s Gal Gadot. In California, at a machine-learning conference late last year, a presenter had to scroll through a sizeable list of white dudes in dark suits before she landed on the first image of a female CEO. It was CEO Barbie.
Data is essential to the operation of an AI system. And the more complicated the system—the more layers in the neural nets, to translate speech or identify faces or calculate the likelihood someone defaults on a loan—the more data must be collected. Programmers might rely on stock photos or Wikipedia entries, archived news articles or audio recordings. They could look at the history of university admissions and parole records. They want clinical studies and credit scores. “Data is very, very important,” says Doina Precup, a professor at McGill’s School of Computer Science. The more data you have, “the better the solution will be.”
But not everyone will be equally represented in that data. Sometimes, this is a function of historical exclusion: In 2017, women represented just 6.4 percent of Fortune 500 CEOs, which is a whopping 52 percent increase over the number from the year before. Health Canada didn’t explicitly request women be included in clinical trials until 1997; according to the Heart and Stroke Foundation’s 2018 Heart Report, two-thirds of heart disease clinical research still focuses on men, which helps explain why a recent study found that symptoms of heart disease are missed in more than half of women. Since we know that women have been kept out of those C-suites and trials, it’s safe to assume that their absence will skew the results of any system trained on this data.
And sometimes, even when ample data exists, those who build the training sets don’t take deliberate measures to ensure its diversity, leading to a facial-recognition program—like the kind Buolamwini needed a novelty mask to fool—that has very different error rates for different groups of people. The result is something called sampling bias, and it’s caused by a dearth of representative data. An algorithm is optimized to make as few mistakes as it possibly can; the goal is to lower its number of errors. But the composition of the data determines where that algorithm directs its attention.
Toniann Pitassi, a University of Toronto computer science professor who focuses on fairness in machine learning, offers the example of a school admission program. (University admissions processes in Canada don’t yet rely on algorithms, Taylor says, but data scientist Cathy O’Neil has found examples of American schools that use them.) “Say you have 5 percent black applicants,” Pitassi says. “If 95 percent of the applicants to that university are white, then almost all your data is going to be white. The algorithm is trying to minimize how many mistakes it makes over all that data, in terms of who should get into the university. But it’s not going to put much effort into minimizing errors in that 5 percent, because it won’t affect the overall error rate.”
“A lot of algorithms are trained by seeing how many answers they get correct in the training data,” explains Suresh Venkatasubramanian, a professor at the University of Utah’s School of Computing. “That’s fine, but if you just count up the answers, there’s a small group that you’re always going to make mistakes on. It won’t hurt you very much to do so, but because you systematically make mistakes on all members of that tiny group, the impact of the erroneous decisions is much more than if your errors were spread out across multiple groups.”
It’s for this reason that Buolamwini still found IBM’s facial-recognition technology to be 87.9 percent accurate. When a system is correct 92.9 percent of the time on the light-skinned women in a data set and 99.7 percent of the time on the light-skinned men, it doesn’t matter that it’s making mistakes on nearly 35 percent of black women. Same with Microsoft’s algorithm, which she found was 93.7 percent accurate at predicting gender. Buolamwini showed that nearly the very same number—93.6 percent—of the gender errors were on the faces of dark-skinned subjects. But the algorithm didn’t need to care.
Spend enough time in deep enough conversation with artificial-intelligence experts, and at some point, they will all offer up the same axiom: garbage in, garbage out. It’s possible to sidestep sampling bias and ensure that systems are being trained on a wealth of balanced data, but if that data comes weighted with our society’s prejudices and discriminations, the algorithm isn’t exactly better off. “What we want is data that is faithful to reality,” Precup says. And when reality is biased, “the algorithm has no choice but to reflect that bias. This is how algorithms are formulated.”
Occasionally, the bias that’s reflected is almost comic in its predictability. Web searches, chat bots, image-captioning programs, and machine translation increasingly rely on a technique called word embeddings. It works by transforming the relationships between words into numerical values, which can let the system mathematically represent language’s social context. Through the technique, an AI system learns the connection between Paris and France and the one between Tokyo and Japan; it perceives the different connection between Tokyo and Paris. In 2016, researchers from Boston University and Microsoft Research fed an algorithm a data set of more than 3 million English words from Google News texts, focused on the ones that were most frequently used, and then prompted it to fill in the blanks. “Man,” they asked, “is to computer programmer as woman is to—what?” The machine shuffled through those words and came back with homemaker.
These statistical correlations are known as latent bias: it’s why an artificial-intelligence institute’s image collection was 68 percent more likely to associate the word cooking with photos of women, and it explains the trouble Google Translate has with languages that use gender-neutral pronouns. A Turkish sentence won’t specify if the doctor is male or female, but the English translation assumes that, if there’s a doctor in the house, he must be a man. This presumption extends to the ads that follow us around the internet. In 2015, researchers found that men were six times more likely than women to be shown Google advertisements for jobs with salaries upwards of $200,000.
The power of the system is its “ability to recognize that correlations occur between gender and professions,” says Kathryn Hume. “The downside is that there’s no intentionality behind the system—it’s just math picking up on correlations. It doesn’t know this is a sensitive issue.” There’s a tension between the futuristic and the archaic at play in this technology. AI is evolving much more rapidly than the data it has to work with, so it’s destined not just to reflect and replicate biases but also to prolong and reinforce them.
Accordingly, groups that have been the target of systemic discrimination by institutions that include police forces and courts don’t fare any better when judgment is handed over to a machine. “The argument that you can produce an AI tool that’s fair and objective is a problem, because you can’t reduce the context in which crime happens to a yes-or-no binary,” says Kelly Hannah-Moffat, a professor at the Centre for Criminology and Sociolegal Studies at the University of Toronto. “We know that race is related to stop-and-frisk policies, carding, and heavier police checking, so if you’re looking at contact with the police or prior arrest rates, you’re looking at a variable that is already biased.” Once that variable is included in the machine-learning system, bias becomes embedded into the algorithmic assessment.
Two years ago, the US investigative news organization ProPublica scrutinized a widely used program known as COMPAS that determines the risk of recidivism in defendants. The reporters collected the scores for more than 7,000 people arrested in a Florida county, then evaluated how many of them had committed crimes in the following two years—the same benchmark used by COMPAS. The algorithm, they discovered, was deeply flawed: the scores were twice as likely to incorrectly flag black defendants for a high risk of recidivism. White defendants who had been labelled low risk, conversely, were nearly twice as likely to be charged with future crimes.
Five states have relied on COMPAS, which disputes ProPublica’s findings, for its criminal-justice algorithm, while other risk-assessment programs are in place in jurisdictions across the country. What’s saved Canada from placing trust in a problematic algorithm might just be our woefully antiquated system. Much of our data “is paper based—our Crown briefs are still done on paper,” Hannah-Moffat says. “So we just don’t have the capacity at a basic technology level to engage in this sort of system.”
To achieve algorithmic impartiality, programmers could simply dispense with attributes like race and gender altogether. But deep-rooted historical correlations—the sort that link women to the kitchen or connect a segregated population with a particular postal code—make it easy for a system to suss out these attributes anyway, even if they’ve been removed. So computer scientists landed on a solution that resembles the orchestral world’s practice of blind auditions: to conceal someone’s identity, they threw up a screen.
“Let’s say we’re concerned about race as the factor of discrimination,” deep-learning pioneer Yoshua Bengio says. “Let’s say we see that, in our data, we can measure race.” Another constraint can be added to the neural network that compels it to ignore information about race, whether that information is implicit, like postal codes, or explicit. That approach can’t create total insensitivity to those protected features, Bengio adds, but it does a pretty good job.
A growing field of research, in fact, now looks to apply algorithmic solutions to the problems of algorithmic bias. This can involve running counterfactuals—having an algorithm analyze what might happen if a woman were approved for a loan, rather than simply combing through what’s happened in the past. It can mean adding constraints to an algorithm, ensuring that when it does make errors those errors are spread equally over every represented group. It’s possible to add a different constraint to the algorithm that lowers the threshold of, say, university acceptance for a particular group, guaranteeing that a representative percentage gets in—call it algorithmic affirmative action.
Still, algorithmic interventions only do so much; addressing bias also demands diversity in the programmers who are training machines in the first place. “It’s not even bad intentions, it’s just that people who do not come from a particular background might be completely oblivious to what that background looks like and how it affects everything,” says McGill professor Doina Precup. Had Joy Buolamwini been in the room when IBM’s data sets were assembled, she could have flagged in an instant that the company’s cutting-edge facial-recognition technology performed abominably on black skin. “What’s at stake when we don’t have a greater understanding of racist and sexist disparities goes far beyond public relations snafus and occasional headlines,” adds Safiya Noble, the author of Algorithms of Oppression. “It means not only are companies missing out on new possibilities for deeper and more diverse consumer engagements, but they’re also likely to not recognize how their products and services are part of systems of power that can be socially damaging.”
A growing awareness of algorithmic bias isn’t only a chance to intervene in our approaches to building AI systems. It’s an opportunity to interrogate why the data we’ve created looks like this and what prejudices continue to shape a society that allows these patterns in the data to emerge. An algorithm, after all, is just a set of instructions. “The algorithms we use are neutral,” Bengio says emphatically. “What is not neutral is the network, once it’s been trained on data that reflects our biases. We are full of biases.”
That’s why we need to be far more mindful about the data we do collect. In March, a Microsoft-led group of researchers came to a conference in San Francisco armed with one possible solution. Because there is no standard way to identify how a data set is created, and no warning label signalling the bias it might contain, they proposed a data sheet that could accompany public data sets and commercial software. The document would make clear where, when, and how the training data was assembled and provide the demographics of subjects used, giving researchers and organizations the necessary information to decide how to use the data sets and in which contexts.
In Europe, a brand-new privacy law called the General Data Protection Regulation includes provisions that restrict the collection of sensitive data, require explanations for algorithmic decision-making, and protect individuals from decisions made about them exclusively by machines. “While these are European laws, it is likely in the long run that Canada will adopt a similar approach in order to be compliant for various trade purposes,” Canada Research Chair Ian Kerr says.
Of course, there’s another solution, elegant in its simplicity and fundamentally fair: get better data. That’s what happened after Joy Buolamwini’s findings chastened IBM for running a facial-recognition system insufficiently balanced for gender and skin tone. The company put together a broader selection of photos to use in its training set. And when IBM tested that new system against images of parliamentarians from countries such as Sweden, Finland, South Africa, and Senegal, something unsurprising happened: the algorithm performed well. For everyone. Not perfectly: the errors on dark-skinned women remained the highest, at 3.46 percent. But it was a ten-fold improvement—more than enough to prove that change is possible, as long as it’s made a priority. Even a halfway intelligent machine knows that.