Listen to an audio version of this story
For more audio from The Walrus, subscribe to AMI-audio podcasts on iTunes.
In the ’90s, when he was a doctoral student at the University of Lausanne, in Switzerland, neuroscientist Sean Hill spent five years studying how cat brains respond to noise. At the time, researchers knew that two regions—the cerebral cortex, which is the outer layer of the brain, and the thalamus, a nut-like structure near the centre—did most of the work. But, when an auditory signal entered the brain through the ear, what happened, specifically? Which parts of the cortex and thalamus did the signal travel to? And in what order? The answers to such questions could help doctors treat hearing loss in humans. So, to learn more, Hill, along with his supervisor and a group of lab techs, anaesthetized cats and inserted electrodes into their brains to monitor what happened when the animals were exposed to sounds, which were piped into their ears via miniature headphones. Hill’s probe then captured the brain signals the noises generated.
The last step was to euthanize the cats and dissect their brains, which was the only way for Hill to verify where he’d put his probes. It was not a part of the study he enjoyed. He’d grown up on a family farm in Maine and had developed a reverence for all sentient life. As an undergraduate student in Massachusetts, he’d experimented on pond snails, but only after ensuring that each was properly anaesthetized. “I particularly loved cats,” he says, “but I also deeply believed in the need for animal data.” (For obvious reasons, neuroscientists cannot euthanize and dissect human subjects.)
Listen to an audio version of this story
For more audio from The Walrus, subscribe to AMI-audio podcasts on iTunes.
Over time, Hill came to wonder if his data was being put to the best possible use. In his cat experiments, he generated reels of magnetic tape—printouts that resembled player piano scrolls. Once he had finished analyzing the tapes, he would pack them up and store them in a basement. “It was just so tangible,” he says. “You’d see all these data coming from the animals, but then what would happen with it? There were boxes and boxes that, in all likelihood, would never be looked at again.” Most researchers wouldn’t even know where to find them.
Hill was coming up against two interrelated problems in neuroscience: data scarcity and data wastage. Over the past five decades, brain research has advanced rapidly—we’ve developed treatments for Parkinson’s and epilepsy and have figured out, if only in the roughest terms, which parts of the brain produce arousal, anger, sadness, and pain—but we’re still at the beginning of the journey. Scientists are still some way, for instance, from knowing the size and shape of each type of neuron (i.e., brain cell), the RNA sequences that govern their behaviour, or the strength and frequency of the electrical signals that pass between them. The human brain has 86 billion neurons. That’s a lot of data to collect and record.
Listen to an audio version of this story
For more audio from The Walrus, subscribe to AMI-audio podcasts on iTunes.
But, while brain data is a precious resource, scientists tend to lock it away, like secretive art collectors. Labs the world over are conducting brain experiments using increasingly sophisticated technology, from hulking magnetic-imaging devices to microscopic probes. These experiments generate results, which then get published in journals. Once each new data set has served this limited purpose, it goes . . . somewhere, typically onto a secure hard drive only a few people can access.
Hill’s graduate work in Lausanne was at times demoralizing. He reasoned that, for his research to be worth the costs to both the lab that conducted it and the cats who were its subjects, the resulting data—perhaps even all brain data—should live in the public domain. But scientists generally prefer not to share. Data, after all, is a kind of currency: it helps generate findings, which lead to jobs, money, and professional recognition. Researchers are loath to simply give away a commodity they worked hard to acquire. “There’s an old joke,” says Hill, “that neuroscientists would rather share toothbrushes than data.”
He believes that, if they don’t get over this aversion—and if they continue to stash data in basements and on encrypted hard drives—many profound questions about the brain will remain unanswered. This is not just a matter of academic curiosity: if we improve our understanding of the brain, we could develop treatments that have long eluded us for major mental illnesses.
In 2019, Hill became director of Toronto’s Krembil Centre for Neuroinformatics (KCNI), an organization working at the intersection of neuroscience, information management, brain modelling, and psychiatry. The basic premise of neuroinformatics is this: the brain is big, and if humans are going to have a shot at understanding it, brain science must become big too. The KCNI’s goal is to aggregate brain data and use it to build computerized models that, over time, become ever more complex—all to aid them in understanding the intricacy of a real brain. There are about thirty labs worldwide explicitly dedicated to such work, and they’re governed by a central regulatory body, the International Neuroinformatics Coordinating Facility, in Sweden. But the KCNI stands out because it’s embedded in a medical institution: the Centre for Addiction and Mental Health (CAMH), Canada’s largest psychiatric hospital. While many other neuroinformatics labs study genetics or cognitive processing, the KCNI seeks to demystify conditions like schizophrenia, anxiety, and dementia. Its first area of focus is depression.
Fundamentally, we don’t have a biological understanding of depression.
The disease affects more than 260 million people around the world, but we barely understand it. We know that the balance between the prefrontal cortex (at the front of the brain) and the anterior cingulate cortex (tucked just behind it) plays some role in regulating mood, as does the chemical serotonin. But what actually causes depression? Is there a tiny but important area of the brain that researchers should focus on? And does there even exist a singular disorder called depression, or is the label a catch-all denoting a bunch of distinct disorders with similar symptoms but different brain mechanisms? “Fundamentally,” says Hill, “we don’t have a biological understanding of depression or any other mental illness.”
The problem, for Hill, requires an ambitious, participatory approach. If neuroscientists are to someday understand the biological mechanisms behind mental illness—that is, if they are to figure out what literally happens in the brain when a person is depressed, manic, or delusional—they will need to pool their resources. “There’s not going to be a single person who figures it all out,” he says. “There’s never going to be an Einstein who solves a set of equations and shouts, ‘I’ve got it!’ The brain is not that kind of beast.”
The KCNI lab has the feeling of a tech firm. It’s an open-concept space with temporary workstations in lieu of offices, and its glassed-in meeting rooms have inspirational names, like “Tranquility” and “Perception.” The KCNI is a “dry centre”: it works with information and software rather than with biological tissue. To obtain data, researchers forge relationships with other scientists and try to convince them to share what they’ve got. The interior design choices are a tactical part of this effort. “The space has to look nice,” says Dan Felsky, a researcher at the centre. “Colleagues from elsewhere must want to come in and collaborate with us.”
Yet it’s hard to forget about the larger surroundings. During one interview in the “Clarity” room, Hill and I heard a code-blue alarm, broadcast across CAMH, to indicate a medical emergency elsewhere in the hospital. Hill’s job doesn’t involve front line care, so he doesn’t personally work with patients, but these disruptions reinforce his sense of urgency. “I come from a discipline where scientists focus on theoretical subjects,” he says. “It’s important to be reminded that people are suffering and we have a responsibility to help them.”
Today, the science of mental illness is based primarily on the study of symptoms. Patients receive a diagnosis when they report or exhibit maladaptive behaviours—despair, anxiety, disordered thinking—associated with a given condition. If a significant number of patients respond positively to a treatment, that treatment is deemed effective. But such data reveals nothing about what physically goes on within the brain. “When it comes to the various diseases of the brain,” says Helena Ledmyr, co-director of the International Neuroinformatics Coordinating Facility, “we know astonishingly little.” Shreejoy Tripathy, a KCNI researcher, gives modern civilization a bit more credit: “The ancient Egyptians would remove the brain when embalming people because they thought it was useless. In theory, we’ve learned a few things since then. In relation to how much we have left to learn, though, we’re not that much further along.”
Joe Herbert, a Cambridge University neuroscientist, offers a revealing comparison between the way mental versus physical maladies are diagnosed. If, in the nineteenth century, you walked into a doctor’s office complaining of shortness of breath, the doctor would likely diagnose you with dyspnea, a word that basically means . . . shortness of breath. Today, of course, the doctor wouldn’t stop there: they would take a blood sample to see if you were anemic, do an X-ray to search for a collapsed lung, or subject you to an echocardiogram to spot signs of heart disease. Instead of applying a Greek label to your symptoms, they’d run tests to figure out what was causing them.
Herbert argues that the way we currently diagnose depression is similar to how we once diagnosed shortness of breath. The term depression is likely as useful now as dyspnea was 150 years ago: it probably denotes a range of wildly different maladies that just happen to have similar effects. “Psychiatrists recognize two types of depression—or three, if you count bipolar—but that’s simply on the basis of symptoms,” says Herbert. “Our history of medicine tells us that defining a disease by its symptoms is highly simplistic and inaccurate.”
The advantage of working with models, as the KCNI researchers do, is that scientists can experiment in ways not possible with human subjects. They can shut off parts of the model brain or alter the electrical circuitry. The disadvantage is that models are not brains. A model is, ultimately, a kind of hypothesis—an illustration, analogy, or computer simulation that attempts to explain or replicate how a certain brain process works. Over the centuries, researchers have created brain models based on pianos, telephones, and computers. Each has some validity—the brain has multiple components working in concert, like the keys of a piano; it has different nodes that communicate with one another, like a telephone network; and it encodes and stores information, like a computer—but none perfectly describes how a real brain works. Models may be useful abstractions, but they are abstractions nevertheless. Yet, because the brain is vast and mysterious and hidden beneath the skull, we have no choice but to model it if we are to study it. Debates over how best to model it, and whether such modelling should be done at the micro or macro scale, are hotly contested in neuroscience. But Hill has spent most of his life preparing to answer these questions.
Hill grew up in the ’70s and ’80s, in an environment entirely unlike the one in which he works. His parents were adherents of the back-to-the-land movement, and his father was an occasional artisanal toymaker. On their farm, near the coast of Maine, the family grew vegetables and raised livestock using techniques not too different from those of nineteenth-century homesteaders. They pulled their plough with oxen and, to fuel their wood-burning stove, felled trees with a manual saw.
When Hill and his older brother found out that the local public school had acquired a TRS-80, an early desktop computer, they became obsessed. The math teacher, sensing their passion, decided to loan the machine to the family for Christmas. Over the holidays, the boys became amateur programmers. Their favourite application was Dancing Demon, in which a devilish figure taps its feet to an old swing tune. Pretty soon, the boys had hacked the program and turned the demon into a monster resembling Boris Karloff in Frankenstein. “In the dark winter of Maine,” says Hill, “what else were we going to do?”
The experiments spurred conversation among the brothers, much of it the fevered speculation of young people who’ve read too much science fiction. They fantasized about the spaceships they would someday design. They also discussed the possibility of building a computerized brain. “I was probably ten or eleven years old,” Hill recalls, “saying to my brother, ‘Will we be able to simulate a neuron? Maybe that’s what we need to get artificial intelligence.’” Roughly a decade later, as an undergraduate at the quirky liberal arts university Hampshire College, Hill was drawn to computational neuroscience, a field whose practitioners were doing what he and his brother had talked about: building mathematical, and sometimes even computerized, brain models.
In 2006, after completing his PhD, along with postgraduate studies in San Diego and Wisconsin, Hill returned to Lausanne to co-direct the Blue Brain Project, a radical brain-modelling lab in the Swiss Alps. The initiative had been founded a year earlier by Henry Markram, a South African Israeli neuroscientist whose outsize ambitions had made him a revered and controversial figure.
In neuroscience today, there are robust debates as to how complex a brain model should be. Some researchers seek to design clean, elegant models. That’s a fitting description of the Nobel Prize–winning work of Alan Hodgkin and Andrew Huxley, who, in 1952, drew handwritten equations and rudimentary illustrations—with lines, symbols, and arrows—describing how electrical signals exit a neuron and travel along a branch-like cable called an axon. Other practitioners seek to make computer-generated maps that incorporate hundreds of neurons and tens of thousands of connections, image fields so complicated that Michelangelo’s Sistine Chapel ceiling looks minimalist by comparison. The clean, simple models demystify brain processes, making them understandable to humans. The complex models are impossible to comprehend: they offer too much information to take in, attempting to approximate the complexity of an actual brain.
Markram’s inclinations are maximalist. In a 2009 TED Talk, he said that he aimed to build a computer model so comprehensive and biologically accurate that it would account for the location and activity of every human neuron. He likened this endeavour to mapping out a rainforest tree by tree. Skeptics wondered whether such a project was feasible. The problem isn’t merely that there are numerous trees in a rainforest: it’s also that each tree has its own configuration of boughs and limbs. The same is true of neurons. Each is a microscopic, blob-like structure with dense networks of protruding branches called axons and dendrites. Neurons use these branches to communicate. Electrical signals run along the axons of one neuron and then jump, over a space called a synapse, to the dendrites of another. The 86 billion neurons in the human brain each have an average of 10,000 synaptic connections. Surely, skeptics argued, it was impossible, using available technology, to make a realistic model from such a complicated, dynamic system.
In 2006, Markram and Hill got to work. The initial goal was to build a hyper-detailed, biologically faithful model of a “microcircuit” (i.e., a cluster of 31,000 neurons) found within the brain of a rat. With a glass probe called a patch clamp, technicians at the lab penetrated a slice of rat brain, connected to each individual neuron, and recorded the electrical signals it sent out. By injecting dye into the neurons, the team could visualize their shape and structure. Step by step, neuron by neuron, they mapped out the entire communication network. They then fed the data into a model so complex that it required Blue Gene, the IBM supercomputer, to run.
In 2015, they completed their rat microcircuit. If they gave their computerized model certain inputs (say, a virtual spark in one part of the circuit), it would predict an output (for instance, an electrical spark elsewhere) that corresponded to biological reality. The model wasn’t doing any actual cognitive processing: it wasn’t a virtual brain, and it certainly wasn’t thinking. But, the researchers argued, it was predicting how electrical signals would move through a real circuit inside a real rat brain. “The digital brain tissue naturally behaves like the real brain tissue,” reads a statement on the Blue Brain Project’s website. “This means one can now study this digital tissue almost like one would study real brain tissue.”
The breakthrough, however, drew fresh criticisms. Some neuroscientists questioned the expense of the undertaking. The team had built a multimillion-dollar computer program to simulate an already existing biological phenomenon, but so what? “The question of ‘What are you trying to explain?’ hadn’t been answered,” says Grace Lindsay, a computational neuroscientist and author of the book Models of the Mind. “A lot of money went into the Blue Brain Project, but without some guiding goal, the whole thing seemed too open ended to be worth the resources.”
Others argued that the experiment was not just profligate but needlessly convoluted. “There are ways to reduce a big system down to a smaller system,” says Adrienne Fairhall, a computational neuroscientist at the University of Washington. “When Boeing was designing airplanes, they didn’t build an entire plane just to figure out how air flows around the wings. They scaled things down because they understood that a small simulation could tell them what they needed to know.” Why seek complexity, she argues, at the expense of clarity and elegance?
The harshest critics questioned whether the model even did what it was supposed to do. When building it, the team had used detailed information about the shape and electrical signals of each neuron. But, when designing the synaptic connections—that is, the specific locations where the branches communicate with one another—they didn’t exactly mimic biological reality, since the technology for such detailed brain mapping didn’t yet exist. (It does now, but it’s a very recent development.) Instead, the team built an algorithm to predict, based on the structure of the neurons and the configuration of the branches, where the synaptic connections were likely to be. If you know the location and shape of the trees, they reasoned, you don’t need to perfectly replicate how the branches intersect.
But Moritz Helmstaedter—a director at the Max Planck Institute for Brain Research, in Frankfurt, Germany, and an outspoken critic of the project—questions whether this supposition is true. “The Blue Brain model includes all kinds of assumptions about synaptic connectivity, but what if those assumptions are wrong?” he asks. The problem, for Helmstaedter, isn’t just that the model could be inaccurate: it’s that there’s no way to fully assess its accuracy given how little we know about brain biology. If a living rat encounters a cat, its brain will generate a flight signal. But, if you present a virtual input representing a cat’s fur to the Blue Brain model, will the model generate a virtual flight signal too? We can’t tell, Helmstaedter argues, in part because we don’t know, in sufficient detail, what a flight signal looks like inside a real rat brain.
Hill takes these comments in stride. To criticisms that the project was too open-ended, he responds that the goal wasn’t to demystify a specific brain process but to develop a new kind of brain modelling based in granular biological detail. The objective, in other words, was to demonstrate—to the world and to funders—that such an undertaking was possible. To criticisms that the model may not work, Hill contends that it has successfully reproduced thousands of experiments on actual rats. Those experiments hardly prove that the simulation is 100 percent accurate—no brain model is—but surely they give it credibility.
And, to criticisms that the model is needlessly complicated, he counters that the brain is complicated too. “We’d been hearing for decades that the brain is too complex to be modelled comprehensively,” says Hill. “Markram put a flag in the ground and said, ‘This is achievable in a finite amount of time.’” The specific length of time is a matter of some speculation. In his TED Talk, Markram implied that he might build a detailed human brain model by 2019, and he began raising money toward a new initiative, the Human Brain Project, meant to realize this goal. But funding dried up, and Markram’s predictions came nowhere close to
panning out.
The Blue Brain Project, however, remains ongoing. (The focus, now, is on modelling a full mouse brain.) For Hill, it offers proof of concept for the broader mission of neuroinformatics. It has demonstrated, he argues, that when you systemize huge amounts of data, you can build platforms that generate reliable insights about the brain. “We showed that you can do incredibly complex data integration,” says Hill, “and the model will give rise to biologically realistic responses.”
When Hill was approached by recruiters on behalf of CAMH to ask if he might consider leaving the Blue Brain Project to start a neuroinformatics lab in Toronto, he demurred. “I’d just become a Swiss citizen,” he says, “and I didn’t want to go.” But the hospital gave him a rare opportunity: to practise cutting-edge neuroscience in a clinical setting. CAMH was formed, in 1998, through a merger of four health care and research institutions. It treats over 34,000 psychiatric patients each year and employs more than 140 scientists, many of whom study the brain. Its mission, therefore, is both psychiatric and neuroscientific—a combination that appealed to Hill. “I’ve spoken to psychiatrists who’ve told me, ‘Neuroscience doesn’t matter,’” he says. “In their work, they don’t think about brain biology. They think about treating the patient in front of them.” Such biases, he argues, reveal a profound gap between brain research and the illnesses that clinicians see daily. At the KCNI, he’d have a chance to bridge that gap.
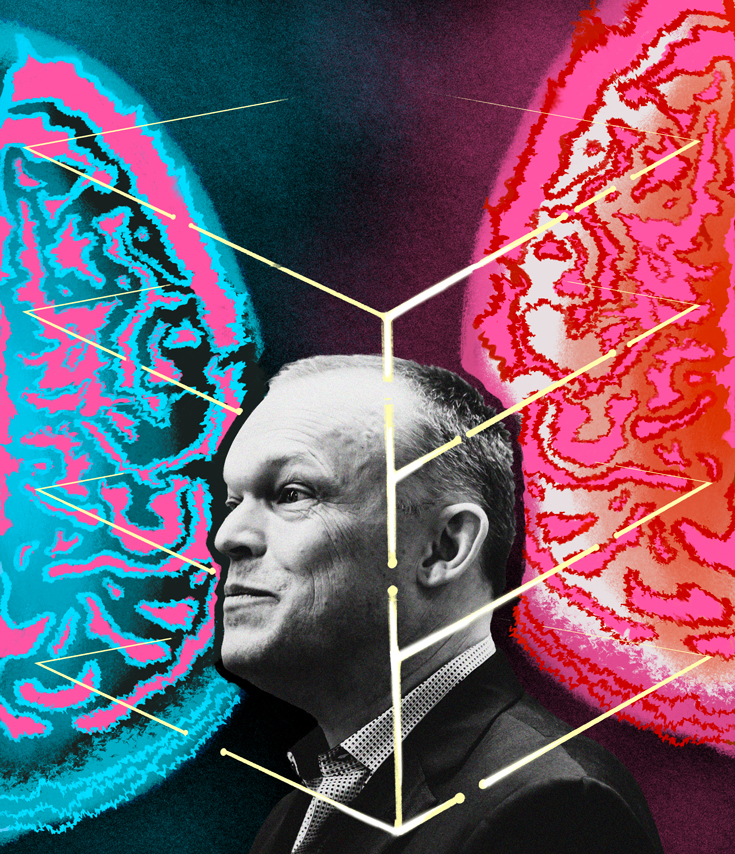
The business of data-gathering and brain-modelling may seem dauntingly abstract, but the goal, ultimately, is to figure out what makes us human. The brain, after all, is the place where our emotional, sensory, and imaginative selves reside. To better understand how the modelling process works, I decided to shadow a researcher and trace an individual data point from its origins in a brain to its incorporation in a KCNI model.
Last February, I met Homeira Moradi, a neuroscientist at Toronto Western Hospital’s Krembil Research Institute who shares data with the KCNI. Because of where she works, she has access to the rarest and most valuable resource in her field: human brain tissue. I joined her at 9 a.m., in her lab on the seventh floor. Below us, on the ground level, Taufik Valiante, a neurosurgeon, was operating on an epileptic patient. To treat epilepsy and brain cancer, surgeons sometimes cut out small portions of the brain. But, to access the damaged regions, they must also remove healthy tissue in the neocortex, the high-functioning outer layer of the brain.
Moradi gets her tissue samples from Valiante’s operating room, and when I met her, she was hard at work weighing and mixing chemicals. The solution in which her tissue would sit would have to mimic, as closely as possible, the temperature and composition of an actual brain. “We have to trick the neurons into thinking they’re still at home,” she said. She moved at the frenetic pace of a line cook during a dinner rush. At some point that morning, Valiante’s assistant would text her from the OR to indicate that the tissue was about to be extracted. When the message came through, she had to be ready. Once the brain sample had been removed from the patient’s head, the neurons within it would begin to die. At best, Moradi would have twelve hours to study the sample before it expired.
The text arrived at noon, by which point we’d been sitting idly for an hour. Suddenly, we sprang into action. To comply with hospital policy, which forbids Moradi from using public hallways where a visitor may spot her carrying a beaker of brains, we approached the OR indirectly, via a warren of underground tunnels. The passages were lined with gurneys and illuminated, like catacombs in an Edgar Allan Poe story, by dim, inconsistent lighting. I hadn’t received permission to witness the operation, so I waited for Moradi outside the OR and was able to see our chunk of brain only once we’d returned to the lab. It didn’t look like much—a marble-size blob, gelatinous and slightly bloody, like gristle on a steak.
Under a microscope, though, the tissue was like nothing I’d ever seen. Moradi chopped the sample into thin pieces, like almond slices, which went into a small chemical bath called a recording chamber. She then brought the chamber into another room, where she kept her “rig”: an infrared microscope attached to a manual arm. She put the bath beneath the lens and used the controls on either side of the rig to operate the arm, which held her patch clamp—a glass pipette with a microscopic tip. On a TV monitor above us, we watched the pipette as it moved through layers of brain tissue resembling an ancient root system—tangled, fibrous, and impossibly dense.
Moradi needed to bring the clamp right up against the wall of a cell. The glass had to fuse with the neuron without puncturing the membrane. Positioning the clamp was maddeningly difficult, like threading the world’s smallest needle. It took her the better part of an hour to connect to a pyramidal neuron, one of the largest and most common cell types in our brain sample. Once we’d made the connection, a filament inside the probe transmitted the electrical signals the neuron sent out. They went first into an amplifier and then into a software application that graphed the currents—strong pulses with intermittent weaker spikes between them—on an adjacent computer screen. “Is that coming from the neuron?” I asked, staring at the screen. “Yes,” Moradi replied. “It’s talking to us.”
A depressive brain is a noisy one. What if scientists could locate the neurons causing the problem?
It had taken us most of the day, but we’d successfully produced a tiny data set—information that may be relevant to the study of mental illness. When neurons receive electrical signals, they often amplify or dampen them before passing them along to adjacent neurons. This function, called gating, enables the brain to select which stimuli to pay attention to. If successive neurons dampen a signal, the signal fades away. If they amplify it, the brain attends more closely. A popular theory of depression holds that the illness has something to do with gating. In depressive patients, neurons may be failing to dampen specific signals, thereby inducing the brain to ruminate unnecessarily on negative thoughts. A depressive brain, according to this theory, is a noisy one. It is failing to properly distinguish between salient and irrelevant stimuli. But what if scientists could locate and analyze a specific cluster of neurons (i.e., a circuit) that was causing the problem?
Etay Hay, an Israeli neuroscientist and one of Hill’s early hires at the KCNI, is attempting to do just that. Using Moradi’s data, he’s building a model of a “canonical” circuit—that is, a circuit that appears thousands of times, with some variations, in the outer layer of the brain. He believes a malfunction in this circuit may underlie some types of treatment-resistant depression. The circuit contains pyramidal neurons, like the one Moradi recorded from, that communicate with smaller cells, called interneurons. The interneurons dampen the signals the pyramidal neurons send them. It’s as if the interneurons are turning down the volume on unwanted thoughts. In a depressive brain, however, the interneurons may be failing to properly reduce the signals, causing the patient to get stuck in negative-thought loops.
Etienne Sibille, another CAMH neuroscientist, has designed a drug that increases communication between the interneurons and the pyramidal neurons in Hay’s circuit. In theory, this drug should enable the interneurons to better do their job, tamp down on negative thoughts, and improve cognitive function. This direct intervention, which occurs at the cellular level, could be more effective than the current class of antidepressants, called SSRIs, which are much cruder. “They take a shotgun approach to depression,” says Sibille, “by flooding the entire brain with serotonin.” (That chemical, for reasons we don’t fully understand, can reduce depressive symptoms, albeit only in some people.)
Sibille’s drug, however, is more targeted. When he gives it to mice who seem listless or fearful, they perk up considerably. Before testing it on humans, Sibille hopes to further verify its efficacy. That’s where Hay comes in. He has finished his virtual circuit and is now preparing to simulate Sibille’s treatment. If the simulation reduces the overall amount of noise in the circuit, the drug can likely proceed to human trials, a potentially game-changing breakthrough.
Hill’s other hires at the KCNI have different specialties from Hay’s but similar goals. Shreejoy Tripathy is building computer models to predict how genes affect the shape and behaviour of neurons. Andreea Diaconescu is using video games to collect data that will allow her to better model early stage psychosis. This can be used to predict symptom severity and provide more effective treatment plans. Joanna Yu is building the BrainHealth Databank, a digital repository for anonymized data—on symptoms, metabolism, medications, and side effects—from over 1,000 CAMH patients with depression. Yu’s team will employ AI to analyze the information and predict which treatment may offer the best outcome for each individual. Similarly, Dan Felsky is helping to run a five-year study on over 300 youth patients at CAMH, incorporating data from brain scans, cognitive tests, and doctors’ assessments. “The purpose,” he says, “is to identify signs that a young person may go on to develop early adult psychosis, one of the most severe manifestations of mental illness.”
All of these researchers are trained scientists, but their work can feel more like engineering: they’re each helping to build the digital infrastructure necessary to interpret the data they bring in.
Sibille’s work, for instance, wouldn’t have been possible without Hay’s computer model, which in turn depends on Moradi’s brain-tissue lab, in Toronto, and on data from hundreds of neuron recordings conducted in Seattle and Amsterdam. This collaborative approach, which is based in data-sharing agreements and trust-based relationships, is incredibly efficient. With a team of three trainees, Hay built his model in a mere twelve months. “If just one lab was generating my data,” he says, “I’d have kept it busy for twenty years.”
Scientific breakthroughs of the past are known to us in part because they lend themselves to storytelling. The tale, probably apocryphal, of Galileo dropping cannonballs from the Tower of Pisa has a protagonist—a freethinker with an unfashionable idea about the world—and a plot twist, whereby an unorthodox theory is suddenly revealed to be true.
The history of neuroscience is filled with similar lore. When, in the 1880s, Santiago Ramón y Cajal, a Spanish researcher, injected dye into brain slices, he established, for once and for all, that brains are made up of neurons (although the term neuron wouldn’t be coined for another few years). And, half a century later, when Wilder Penfield, a Montreal epilepsy surgeon, administered shocks to his patients’ brains, conjuring physical sensations in their bodies (such as the famous burnt-toast discovery) and hallucinations in their minds, he demonstrated that sensory experiences arise from electrical stimuli. These simple narratives are themselves a kind of analogy, or model. We hear them, and suddenly, we better understand our world.
But the remaining neuroscientific questions, ones about the nature of consciousness or the molecular origins of mental illness, are so complex that they demand a depersonalized type of science with greater feats of digital engineering, fewer simple experiments, and only incremental changes in understanding. To this end, the KCNI aims to make all its models open so that other labs can combine them with models of their own, creating bigger, more comprehensive wholes. “Each lab can have its wins by building individual pieces,” says Hill. “But, over the years, the entire project must become bigger than the sum of its parts.”
This approach presents its share of challenges. One is getting scientists to work together despite the academic market’s incentives to the contrary. The university system—where researchers hoard data for fear that it may fall into the hands of competitors—is a major obstacle to cooperation. So, too, is the academic publishing industry, which favours novel findings over raw data. Hill believes that data journals, a niche genre, should become every bit as prestigious as their most esteemed peers (like The Lancet or The New England Journal of Medicine) and that university hiring and tenure committees should reward applicants not only for the number of studies they publish but also for their contributions to the collective storehouse of knowledge. To get hired at the KCNI, candidates must demonstrate a commitment to collaborative science. Hill is working with colleagues at CAMH to instill similar standards hospital wide, and he hopes, in time, to advise the University of Toronto on its hiring and promotion practices.
It’s as if we’re studying random stills from a movie but not seeing the entire thing.
Encouragingly for Hill, funders are slowly getting on board with collaborative neuroscience—not just the Krembil Foundation, which backs the KCNI, but also the US National Institutes of Health, which has supported data-sharing platforms in neuroscience, and Seattle’s Allen Institute for Brain Science, founded by deceased Microsoft cofounder Paul Allen, which is among the biggest producers of publicly available brain data. In support of such work, Hill is currently leading an international team to figure out common standards to describe data sets so they can be formatted and shared easily. Without such new incentives and new ways of practising science, we’ll never be able to properly study mental illness. We know, for instance, that mental disorders can morph into one another—a bout of anxiety can become a depressive episode and then a psychotic break, each phase a step on a timeline—but we cannot understand this timeline unless we build models to simulate how illnesses mutate inside the brain. “Right now,” says Hill, “it’s as if we’re studying random stills from a movie but not seeing the entire thing.”
Seeing the entire thing is the point of neuroinformatics. But, Hill argues, this obsession with bigness also makes it different from much of the brain research that came before. “Science is about extracting information from reality,” says Fairhall, of the University of Washington. “Reality has lots of parameters, but the goal of science is to boil it down to the bits that matter.” It is, by definition, the process of distilling our complicated world to a set of comprehensible principles.
Yet this definition poses a problem for neuroscience. Because the brain remains largely unmapped and unexplored, researchers don’t yet have access to the reality they’re trying to distill. If they are to reduce the brain to a set of understandable rules, they must first engineer a better composite picture of the brain itself. That’s why, for Hill, brain science must pursue bigness and complexity before it can derive the simple, elegant solutions that are its ultimate objective. Its goal, for now, shouldn’t be to elevate individual scientists to celebrity status or to tell stories that rival those of Cajal or Penfield. Rather, it should be to build—over time and with the aid of a global community—a data machine so complex that it can help us model the equally formidable machines that reside within our heads.
Such ambitious work may seem abstract, but it can yield moments of profound poetry. One of Hill’s favourite recent experiments came not from neuroscience but from astronomy—a discipline, popularized by ’60s icons like Carl Sagan, that has a hippie ethos and has been more open, historically, to cooperative science. In 2019, a group of 200 researchers pooled enough data from telescopes in Chile, Mexico, Antarctica, and the United States to fill 1,000 hard drives and produce a composite photograph of the black hole at the centre of the Messier 87 galaxy. It was an unprecedented achievement. The Messier 87 galaxy is 54 million light years from Earth, too far for any single telescope to capture. Yet the experimenters showed that, when members of a scientific community throw their collective weight against a single hard problem, they can push the boundaries of what’s knowable. “They turned the planet into a telescope,” Hill says admiringly. “Now, we must turn it into a brain.”
Correction May 5, 2022: An earlier version of this article stated that Hampshire College is in New Hampshire. In fact, it is in Amherst, Massachusetts. The Walrus regrets the error.
June 2, 2021: A previous version of this story stated that a composite photograph of Sagittarius A* was released in 2019, that one of the telescopes used in this project was located in France, and that the black hole in the image was 26,000 light years from Earth. In fact, the image was of the black hole at the centre of the Messier 87 galaxy, none of the telescopes used were in France, and the Messier 87 galaxy is 54 million light years from Earth. The Walrus regrets the errors.